Interpretable machine learning for the identification of estrogen receptor agonists, antagonists, and binders - a tool for identifying risks from chemicals
Uko Maran
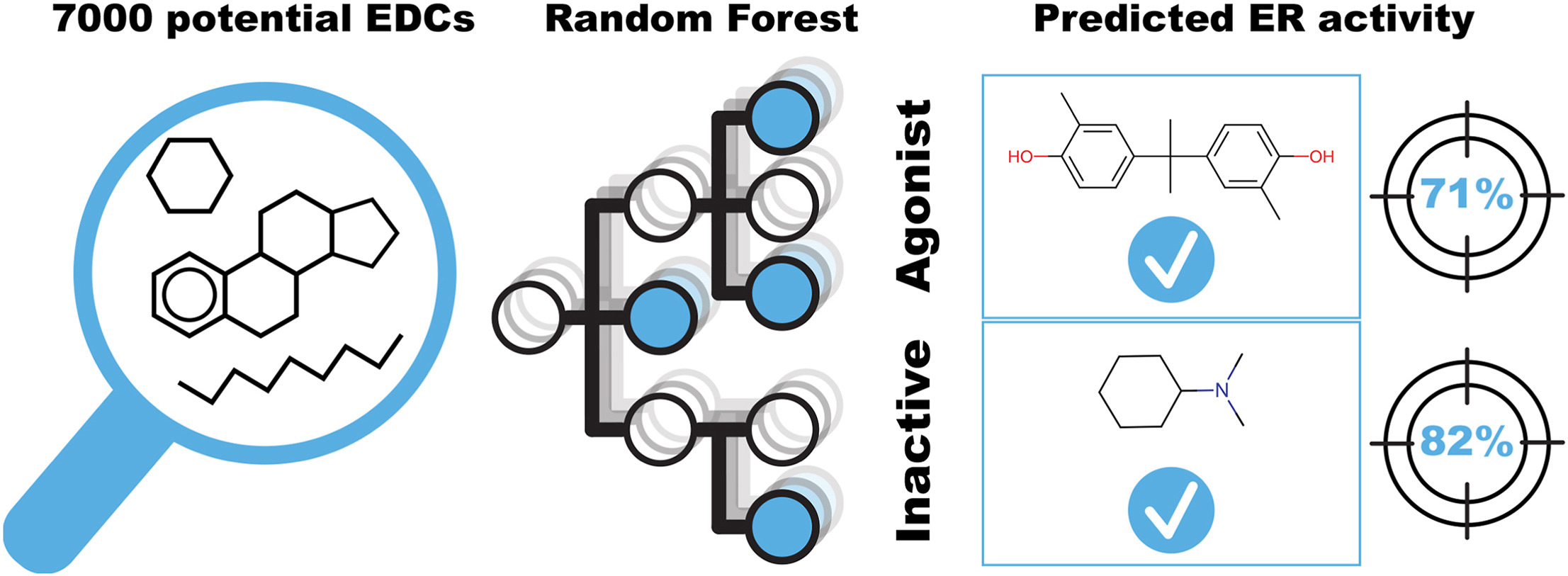
An abnormal hormonal activity or exposure to endocrine-disrupting chemicals (EDCs) can cause endocrine system malfunction. Among the many interactions EDCs can affect is the disruption of estrogen signaling, which can lead to adverse health effects such as cancer, osteoporosis, neurodegenerative diseases, cardiovascular disease, insulin resistance, and obesity. Knowing which chemical can act as an EDC is a significant advantage and a practical necessity. New Approach Methodologies (NAM) computational models offer a quick and cost-effective solution for preliminary hazard assessment of chemicals without animal testing. Therefore, a machine learning approach was used to investigate the relationships between estrogen receptor (ER) activity and chemical structure to identify chemicals that can interact with ER. For this purpose, the consolidated in vitro assay data from ToxCast/Tox21 projects was used for developing Random Forest classification models for ER binding, agonists, and antagonists. The overall classification prediction accuracy reaches up to 82%, depending on whether the model predicted agonists, antagonists, or compounds that bind to the active site. Given the imbalance in endocrine disruption data, the derived models are good candidates for deprioritising chemicals and reducing animal testing. The interpretation of theoretical molecular descriptors of the models was consistent with the molecular interactions known in the ligand binding pocket. The estimated class probabilities enabled the analysis of the applicability domain of the developed models and the assessment of the predictions’ reliability, followed by the guidelines for interpreting prediction results. The models are openly accessible and useable at QsarDB.org according to the FAIR (Findable, Accessible, Interoperable, Reusable) principles.
Article: https://doi.org/10.1016/j.chemosphere.2023.140671
FAIR data and model: http://dx.doi.org/10.15152/QDB.259